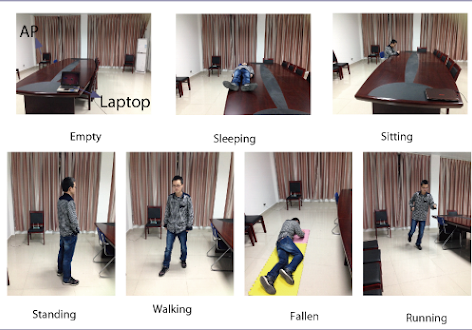
Humans and their activities have long been labeled as obstacles and disturbances for wireless ambient signals. However, only till recently people realize that the signal fluctuation caused by human beings is an enriching source for detecting human motions. The central idea is that signals in a silence'' environment serve well as a
golden’’ benchmark for recognizing motions that lead to signal variances.Therefore, exploring ambient signals for detecting motions emerges as an enabling technology. Though significant progress has been achieved and some pioneering prototypes have been built, there still exist certain open issues calling for immediate attention, e.g., theoretical analysis and modelling between human motions and signal variances in terms of channel response. Though tremendous efforts have been devoted and significant progresses have been achieved, there still exist certain open issues calling for immediate attention, e.g., theoretical modelling between human motions and signal variances.
This research fills in the void by presenting a systematic view of the channel response based motion detection problem. Via mathematical analysis, we offer fundamental understandings on the relationship between human motions and channel response, including a sensitivity analysis model. Based on this model, we propose a Low-overhead and real-time Motion Detection algorithm (LoMD), which leverages signals in a ``silence’’ environment as a reference for distinguishing motions causing signal changes.
To validate our algorithm, we build a prototype on which extensive real-world experiments have been conducted. By comparing LoMD with another state-of-the-art method, i.e., FIMD, we have shown that LoMD outperforms FIMD in terms of computational complexity, detection accuracy and false alarm rate. On average, LoMD can achieve detection accuracy as high as 97.38% and false negative rate as low as 6.33%.